[ad_1]
Analysis within the area of machine studying and AI, now a key know-how in virtually each business and firm, is way too voluminous for anybody to learn all of it. This column, Perceptron, goals to gather among the most related latest discoveries and papers — significantly in, however not restricted to, synthetic intelligence — and clarify why they matter.
An “earable” that makes use of sonar to learn facial expressions was among the many initiatives that caught our eyes over these previous few weeks. So did ProcTHOR, a framework from the Allen Institute for AI (AI2) that procedurally generates environments that can be utilized to coach real-world robots. Among the many different highlights, Meta created an AI system that may predict a protein’s construction given a single amino acid sequence. And researchers at MIT developed new hardware that they declare gives quicker computation for AI with much less vitality.
The “earable,” which was developed by a staff at Cornell, seems one thing like a pair of cumbersome headphones. Audio system ship acoustic alerts to the aspect of a wearer’s face, whereas a microphone picks up the barely-detectable echoes created by the nostril, lips, eyes, and different facial options. These “echo profiles” allow the earable to seize actions like eyebrows elevating and eyes darting, which an AI algorithm interprets into full facial expressions.
Picture Credit: Cornell
The earable has a number of limitations. It solely lasts three hours on battery and has to dump processing to a smartphone, and the echo-translating AI algorithm should practice on 32 minutes of facial knowledge earlier than it will possibly start recognizing expressions. However the researchers make the case that it’s a a lot sleeker expertise than the recorders historically utilized in animations for motion pictures, TV, and video video games. For instance, for the thriller recreation L.A. Noire, Rockstar Video games constructed a rig with 32 cameras skilled on every actor’s face.
Maybe sometime, Cornell’s earable will probably be used to create animations for humanoid robots. However these robots should learn to navigate a room first. Fortuitously, AI2’s ProcTHOR takes a step (no pun meant) on this course, creating hundreds of customized scenes together with lecture rooms, libraries, and workplaces by which simulated robots should full duties, like selecting up objects and shifting round furnishings.
The concept behind the scenes, which have simulated lighting and include a subset of an enormous array of floor supplies (e.g., wooden, tile, and so on.) and family objects, is to show the simulated robots to as a lot selection as doable. It’s a well-established idea in AI that efficiency in simulated environments can enhance the efficiency of real-world techniques; autonomous automotive corporations like Alphabet’s Waymo simulate total neighborhoods to fine-tune how their real-world vehicles behave.
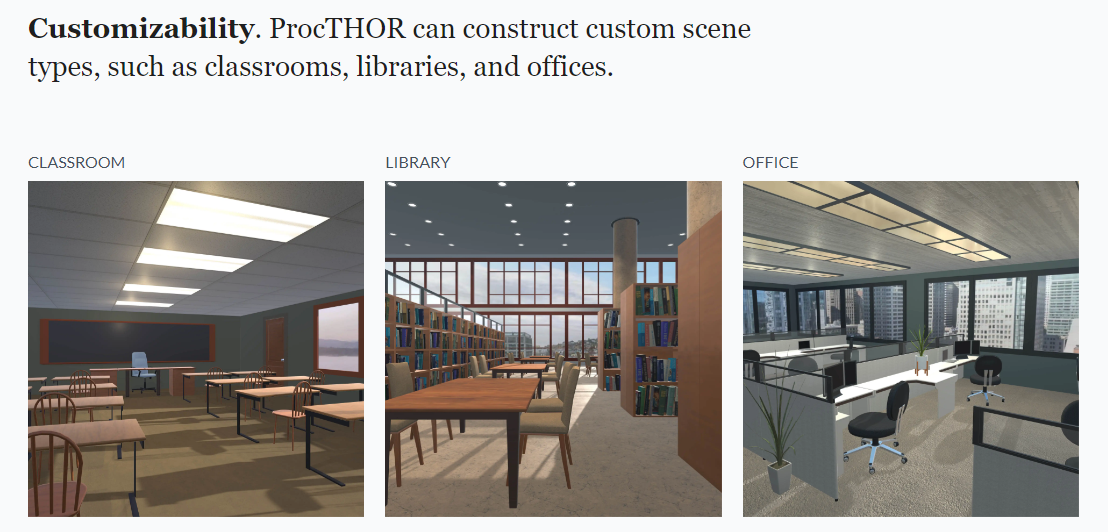
Picture Credit: Allen Institute for Synthetic Intelligence
As for ProcTHOR, AI2 claims in a paper that scaling the variety of coaching environments constantly improves efficiency. That bodes effectively for robots sure for properties, workplaces, and elsewhere.
In fact, coaching these kind of techniques requires a whole lot of compute energy. However which may not be the case perpetually. Researchers at MIT say they’ve created an “analog” processor that can be utilized to create superfast networks of “neurons” and “synapses,” which in flip can be utilized to carry out duties like recognizing pictures, translating languages, and extra.
The researchers’ processor makes use of “protonic programmable resistors” organized in an array to “be taught” expertise. Growing and reducing {the electrical} conductance of the resistors mimics the strengthening and weakening of synapses between neurons within the mind, part of the educational course of.
The conductance is managed by an electrolyte that governs the motion of protons. When extra protons are pushed right into a channel within the resistor, the conductance will increase. When protons are eliminated, the conductance decreases.
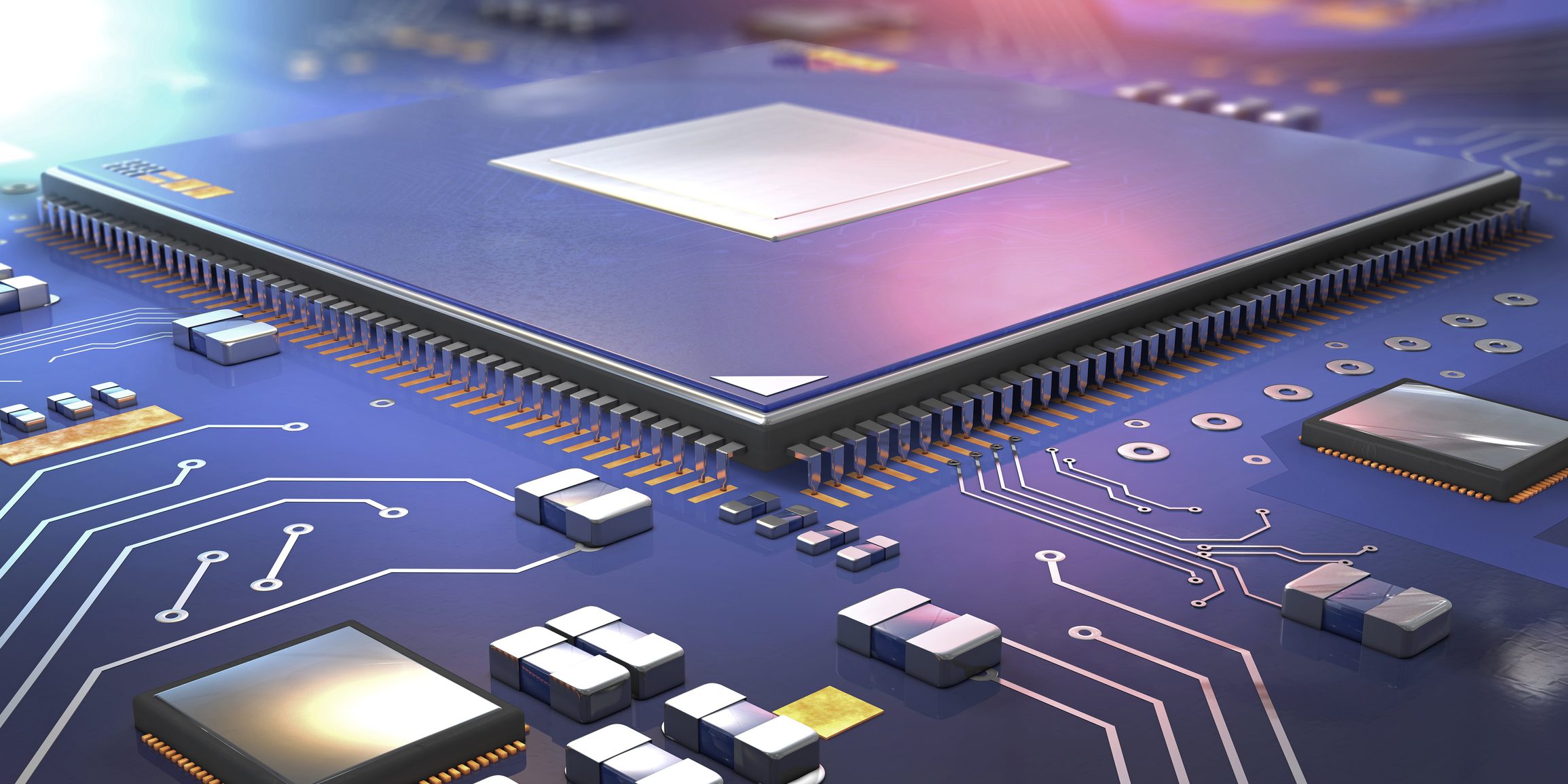
Processor on a pc circuit board
An inorganic materials, phosphosilicate glass, makes the MIT staff’s processor extraordinarily quick as a result of it comprises nanometer-sized pores whose surfaces present the right paths for protein diffusion. As an additional benefit, the glass can run at room temperature, and it isn’t broken by the proteins as they transfer alongside the pores.
“After you have an analog processor, you’ll now not be coaching networks everybody else is engaged on,” lead writer and MIT postdoc Murat Onen was quoted as saying in a press launch. “You may be coaching networks with unprecedented complexities that nobody else can afford to, and due to this fact vastly outperform all of them. In different phrases, this isn’t a quicker automotive, this can be a spacecraft.”
Talking of acceleration, machine studying is now being put to make use of managing particle accelerators, at least in experimental form. At Lawrence Berkeley Nationwide Lab two groups have proven that ML-based simulation of the complete machine and beam offers them a extremely exact prediction as a lot as 10 instances higher than odd statistical evaluation.
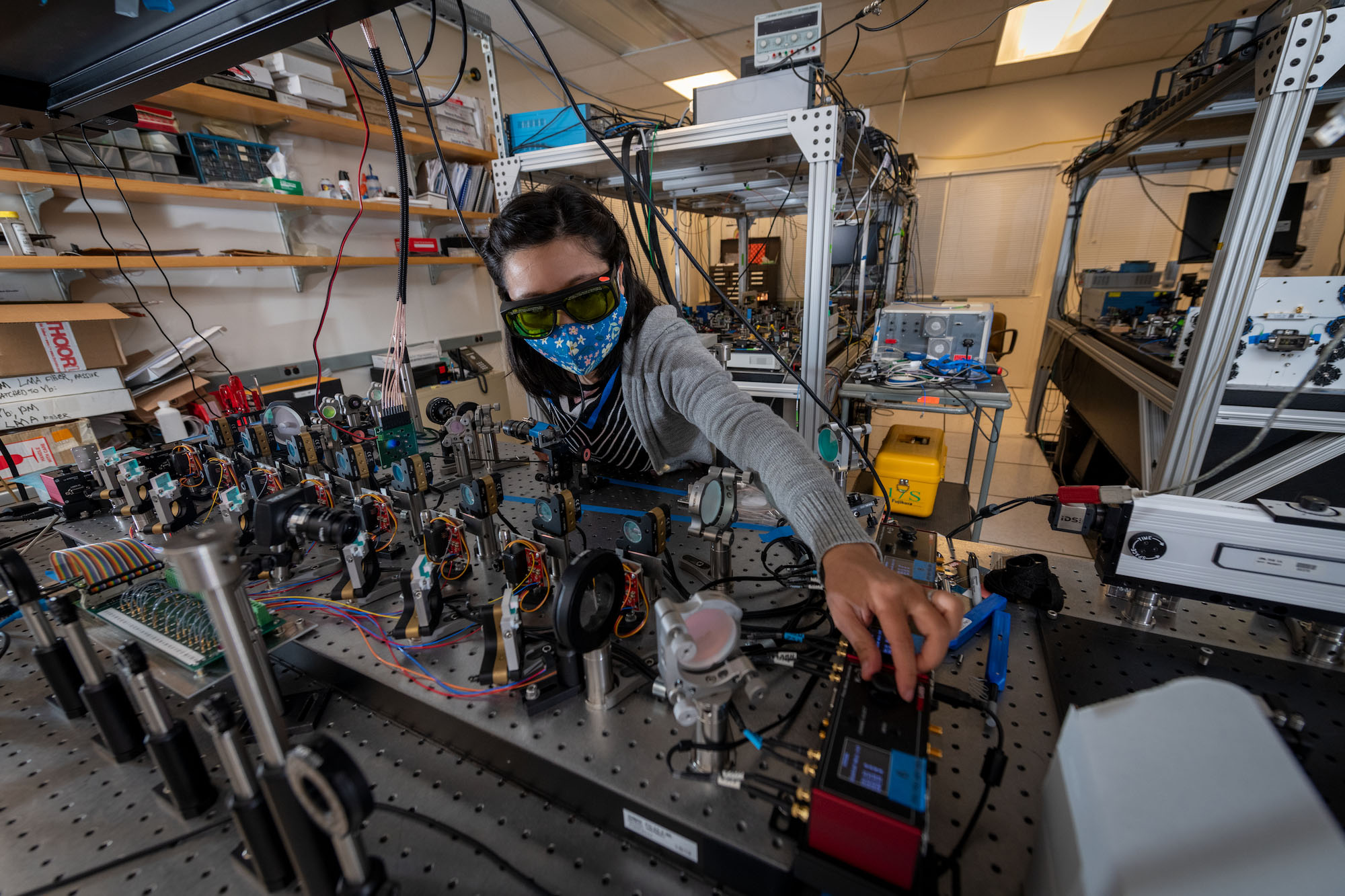
Picture Credit: Thor Swift/Berkeley Lab
“In case you can predict the beam properties with an accuracy that surpasses their fluctuations, you’ll be able to then use the prediction to extend the efficiency of the accelerator,” mentioned the lab’s Daniele Filippetto. It’s no small feat to simulate all of the physics and gear concerned, however surprisingly the assorted groups’ early efforts to take action yielded promising outcomes.
And over at Oak Ridge Nationwide Lab an AI-powered platform is letting them do Hyperspectral Computed Tomography utilizing neutron scattering, discovering optimum… maybe we should just let them explain.
Within the medical world, there’s a brand new utility of machine learning-based picture evaluation within the area of neurology, the place researchers at College School London have skilled a mannequin to detect early signs of epilepsy-causing brain lesions.
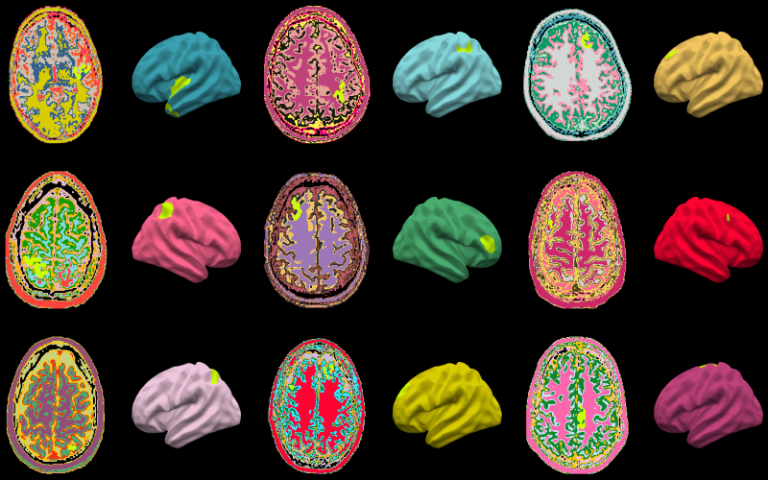
MRIs of brains used to coach the UCL algorithm.
One frequent reason behind drug-resistant epilepsy is what is named a focal cortical dysplasia, a area of the mind that has developed abnormally however for no matter motive doesn’t seem clearly irregular in MRI. Detecting it early could be extraordinarily useful, so the UCL staff skilled an MRI inspection mannequin referred to as Multicentre Epilepsy Lesion Detection on hundreds of examples of wholesome and FCD-affected mind areas.
The mannequin was capable of detect two thirds of the FCDs it was proven, which is definitely fairly good because the indicators are very delicate. Actually, it discovered 178 circumstances the place medical doctors had been unable to find an FCD however it may. Naturally the ultimate say goes to the specialists, however a pc hinting that one thing is likely to be improper can generally be all it takes to look nearer and get a assured prognosis.
“We put an emphasis on creating an AI algorithm that was interpretable and will assist medical doctors make selections. Displaying medical doctors how the MELD algorithm made its predictions was a necessary a part of that course of,” mentioned UCL’s Mathilde Ripart.
Source link